Data governance principles are the bedrock of effective information management in modern organizations. As businesses amass vast quantities of data, these principles serve as crucial guidelines for ensuring its accuracy, security, and ethical use.
They encompass a range of practices that help companies extract maximum value from their information assets while maintaining compliance with evolving regulations.
This guide looks at the core data governance principles, exploring how they can transform raw data into a strategic resource. We’ll examine practical implementation strategies, common challenges, and the long-term benefits of adopting a robust data governance framework.
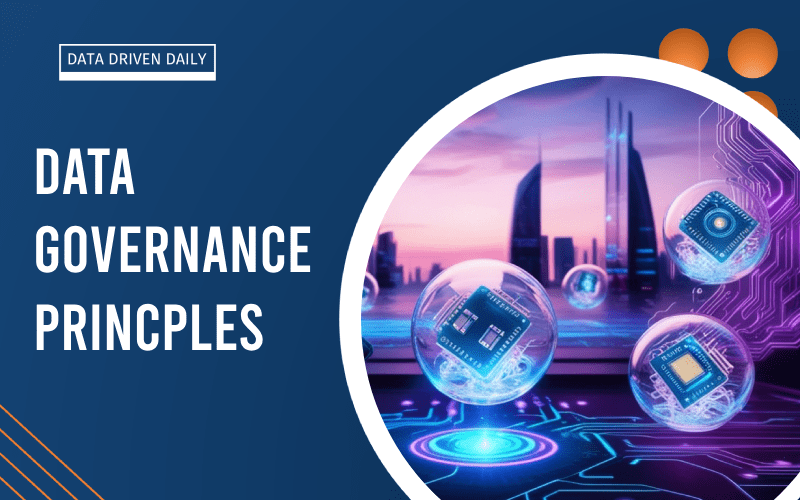
What Are Data Governance Principles?
Data governance principles form the bedrock of how organizations handle their information assets. These guidelines encompass a wide range of practices and strategies aimed at ensuring data is accurate, secure, accessible, and compliant with relevant regulations.
Key Aspects of Data Governance:
- Data quality management
- Information security protocols
- Privacy protection measures
- Regulatory compliance frameworks
- Data accessibility standards
- Lifecycle management strategies
- Metadata management practices
- Data integration and interoperability
- Risk management and mitigation
- Data ethics and responsible use
The Critical Importance of Data Governance
Implementing robust data governance isn’t just about ticking boxes—it’s about creating tangible value for your organization. Here’s why it matters:
- Enhanced Decision-Making: With clean, reliable data at their fingertips, leaders can make informed choices based on accurate insights.
- Risk Mitigation: Proper governance significantly reduces the likelihood of data breaches, regulatory fines, and reputational damage.
- Operational Efficiency: Streamlined data processes lead to faster, more effective operations across the board.
- Increased Trust: Customers, partners, and stakeholders have greater confidence in organizations that handle data responsibly.
- Innovation Catalyst: Well-governed data can spark new ideas and drive product or service innovations.
- Cost Savings: By eliminating redundancies and improving data quality, organizations can reduce data-related expenses.
- Competitive Advantage: In a data-driven world, companies with superior data governance often outperform their peers.
7 Essential Data Governance Principles: In-Depth Exploration
Let’s dive deep into the core principles that form the foundation of effective data governance:
1. Data Ownership and Stewardship
Every piece of data within an organization should have a clearly defined owner who’s responsible for its management, quality, and appropriate use.
Implementing Data Ownership:
- Create a Data Governance Council: This cross-functional team oversees the organization’s data strategy.
- Define Roles and Responsibilities: Clearly outline the duties of data owners, stewards, and custodians.
- Develop an Ownership Matrix: Map data assets to their respective owners across departments.
- Implement Accountability Measures: Set KPIs for data owners to ensure they’re actively managing their assets.
- Provide Training: Equip data owners with the skills and knowledge they need to fulfill their responsibilities.
💡 Tip: Consider implementing a RACI (Responsible, Accountable, Consulted, Informed) matrix for data ownership to clarify roles and responsibilities.
2. Data Quality and Integrity
Ensuring the accuracy, completeness, and consistency of data is paramount for reliable decision-making and operational efficiency.
Comprehensive Data Quality Checklist:
- Accuracy: Data reflects real-world values and is free from errors
- Completeness: All required data fields are populated without missing or null values
- Consistency: Data is uniform across all systems and databases
- Timeliness: Data is up-to-date and reflects the most current information
- Validity: Data adheres to defined formats, types, and value ranges
- Uniqueness: No unnecessary duplication of data entries
- Relevance: Data is applicable and helpful for the intended use case
- Accessibility: Authorized users can easily retrieve the data when needed
- Conformity: Data adheres to standard formats and conventions
- Precision: Data has the right level of detail for its intended use
Strategies for Improving Data Quality:
- Implement Data Profiling: Regularly analyze data to identify quality issues.
- Set Up Data Validation Rules: Create automated checks to catch errors at the point of entry.
- Conduct Regular Audits: Perform systematic reviews of data quality across systems.
- Use Master Data Management (MDM): Implement MDM solutions to ensure consistency across the organization.
- Automate Data Cleansing: Use tools to automatically correct common data quality issues.
- Provide Data Quality Training: Educate employees on the importance of data quality and best practices.
3. Data Security and Privacy
Protecting sensitive information from unauthorized access, breaches, and misuse is critical in today’s threat landscape.
Comprehensive Security Measures:
- Encryption: Implement strong encryption for data at rest and in transit.
- Access Controls: Use role-based access control (RBAC) and principle of least privilege.
- Regular Security Audits: Conduct frequent assessments of your security posture.
- Employee Training: Provide ongoing education on data handling and security best practices.
- Incident Response Plans: Develop and regularly test plans for responding to data breaches.
- Network Segmentation: Isolate sensitive data on separate network segments.
- Multi-Factor Authentication (MFA): Require MFA for accessing critical data systems.
- Data Loss Prevention (DLP): Implement DLP tools to prevent unauthorized data exfiltration.
- Vulnerability Management: Regularly scan for and patch security vulnerabilities.
- Physical Security: Ensure physical access to data centers and servers is strictly controlled.
Privacy Protection Strategies:
- Implement data minimization practices
- Use data anonymization and pseudonymization techniques
- Conduct regular privacy impact assessments
- Develop clear data collection and usage policies
- Provide easy-to-use privacy controls for customers
- Appoint a dedicated Data Protection Officer (DPO)
4. Regulatory Compliance
Adhering to relevant laws and regulations governing data usage is non-negotiable in today’s regulatory environment.
Key Data Regulations Table:
Regulation | Region | Focus |
GDPR | European Union | Data protection and privacy |
CCPA | California, USA | Consumer privacy rights |
HIPAA | United States | Healthcare data protection |
PIPEDA | Canada | Personal information protection |
Compliance Best Practices:
- Conduct Regular Compliance Audits: Systematically review your data practices against regulatory requirements.
- Maintain Detailed Documentation: Keep comprehensive records of data processing activities and compliance efforts.
- Implement Data Discovery Tools: Use automated solutions to identify and classify regulated data across your systems.
- Develop a Compliance Training Program: Regularly educate employees on relevant regulations and compliance procedures.
- Create Data Retention and Deletion Policies: Ensure data is not kept longer than necessary and is securely disposed of when no longer needed.
- Establish a Breach Notification Process: Develop procedures for timely notification of authorities and affected individuals in case of a data breach.
- Conduct Data Protection Impact Assessments (DPIAs): Regularly assess the privacy risks associated with new data processing activities.
5. Data Accessibility and Sharing
Striking the right balance between data access and security is crucial for maximizing the value of your data assets while minimizing risks.
Best Practices for Data Accessibility:
- Implement role-based access control (RBAC) systems
- Create clear data sharing agreements and policies
- Use secure file transfer protocols for data exchanges
- Maintain comprehensive audit logs of data access and sharing
- Develop a data catalog to improve discoverability
- Implement self-service data access tools for authorized users
- Regularly review and update access permissions
Data Sharing Framework:
- Internal Sharing:
- Implement cross-departmental data sharing protocols
- Use collaborative platforms for secure data sharing
- Establish clear guidelines for internal data usage
- External Sharing:
- Develop robust data sharing agreements with partners
- Use secure APIs for controlled data access
- Implement data anonymization for sensitive information
- Public Data Sharing:
- Identify datasets suitable for public release
- Ensure proper data cleansing and anonymization
- Use open data platforms for public data dissemination
🔑 Key Consideration: Always balance the need for data accessibility with security and privacy requirements.
6. Data Lifecycle Management
Managing data effectively throughout its entire lifecycle ensures it remains useful, compliant, and secure from creation to deletion.
Detailed Data Lifecycle Stages:
- Creation/Acquisition:
- Implement data quality checks at the point of entry
- Ensure proper metadata tagging
- Verify data source reliability
- Storage:
- Choose appropriate storage solutions based on data type and usage
- Implement data encryption and access controls
- Regularly back up data and test restore procedures
- Usage:
- Monitor data access and usage patterns
- Implement data lineage tracking
- Ensure compliance with usage policies
- Sharing:
- Use secure data transfer methods
- Implement data masking for sensitive information
- Maintain detailed logs of all data sharing activities
- Archiving:
- Develop clear archiving criteria and schedules
- Use appropriate archiving technologies
- Ensure archived data remains accessible when needed
- Deletion:
- Implement secure data deletion procedures
- Maintain deletion logs for compliance purposes
- Regularly review and enforce data retention policies
Data Lifecycle Management Strategies:
- Develop a comprehensive data lifecycle policy
- Use data lifecycle management tools to automate processes
- Regularly audit data to ensure it’s in the appropriate lifecycle stage
- Train employees on proper data handling at each lifecycle stage
- Implement data classification to guide lifecycle management decisions
7. Metadata Management
Maintaining comprehensive information about your data assets improves discoverability, understanding, and proper usage of data across the organization.
Key Metadata Elements:
- Descriptive Metadata: Title, description, keywords, creator, creation date
- Structural Metadata: File type, size, relationships to other data
- Administrative Metadata: Access permissions, retention period, data owner
- Technical Metadata: Format, encoding, software requirements
- Business Metadata: Business context, usage guidelines, data quality scores
- Operational Metadata: Last update time, access frequency, data lineage
Metadata Management Best Practices:
- Implement a Metadata Repository: Create a centralized system for storing and managing metadata.
- Automate Metadata Capture: Use tools to automatically extract and update metadata.
- Develop Metadata Standards: Create organization-wide standards for metadata creation and management.
- Integrate with Data Catalogs: Link metadata to your data catalog for improved discoverability.
- Regular Metadata Audits: Periodically review and update metadata to ensure accuracy.
- Metadata Governance: Establish clear roles and responsibilities for metadata management.
- User-Friendly Interfaces: Provide easy-to-use tools for metadata creation and access.
Implementing Data Governance Principles: A Comprehensive Approach
Putting these principles into practice requires a structured, organization-wide approach:
- Assess Current State:
- Conduct a thorough data inventory
- Evaluate existing data management practices
- Identify gaps and areas for improvement
- Define Objectives:
- Set clear, measurable goals for your data governance program
- Align objectives with overall business strategy
- Prioritize initiatives based on business impact and feasibility
- Develop Policies and Standards:
- Create comprehensive data governance policies
- Develop data standards and guidelines
- Ensure alignment with regulatory requirements
- Assign Roles and Responsibilities:
- Establish a data governance team
- Define clear roles for data owners, stewards, and custodians
- Create a RACI matrix for data-related activities
- Implement Tools and Technologies:
- Deploy data governance software solutions
- Implement data quality and metadata management tools
- Integrate data governance capabilities into existing systems
- Train Personnel:
- Develop a comprehensive data governance training program
- Provide role-specific training for data stewards and owners
- Conduct organization-wide awareness sessions
- Monitor and Measure:
- Define key performance indicators (KPIs) for data governance
- Implement monitoring and reporting tools
- Regularly review progress and adjust strategies as needed
- Continuous Improvement:
- Solicit feedback from stakeholders
- Stay updated on industry best practices and emerging technologies
- Regularly reassess and refine your data governance program
🔑 Success Factor: Strong leadership support and a culture of data-driven decision-making are crucial for effective data governance implementation.
Overcoming Data Governance Challenges: Strategies for Success
Implementing data governance principles can be a complex undertaking. Here are some common challenges and strategies to overcome them:
Challenge 1: Resistance to Change
Many organizations face pushback when implementing new data governance practices.
Solutions:
- Clearly communicate the benefits of data governance to all stakeholders
- Involve key personnel early in the planning process
- Showcase quick wins to build momentum
- Provide ample training and support during the transition
- Address concerns and objections proactively
Challenge 2: Lack of Resources
Data governance initiatives often compete for limited budget and personnel.
Solutions:
- Start with small, high-impact pilot projects to demonstrate ROI
- Leverage existing tools and technologies where possible
- Consider a phased approach to implementation
- Build a strong business case highlighting long-term benefits
- Explore partnerships with external data governance experts
Challenge 3: Siloed Data and Processes
Many organizations struggle with disconnected data systems and departmental silos.
Solutions:
- Promote cross-departmental collaboration through joint initiatives
- Implement data integration technologies to connect disparate systems
- Develop organization-wide data standards and protocols
- Create cross-functional data governance teams
- Use data catalogs and metadata management to improve data discoverability
Challenge 4: Keeping Up with Evolving Regulations
The regulatory landscape is constantly changing, making compliance a moving target.
Solutions:
- Partner with legal experts to stay informed about regulatory changes
- Invest in compliance management tools and technologies
- Develop a flexible data governance framework that can adapt to new requirements
- Participate in industry associations and forums to share best practices
- Conduct regular compliance audits and assessments
Challenge 5: Measuring Data Governance Success
Quantifying the impact of data governance initiatives can be challenging.
Solutions:
- Define clear, measurable KPIs for your data governance program
- Implement tools to track data quality, usage, and governance metrics
- Conduct regular surveys to assess stakeholder satisfaction
- Use case studies and success stories to illustrate qualitative benefits
- Tie data governance metrics to broader business outcomes
The Future of Data Governance: Emerging Trends and Technologies
As technology continues to advance, data governance principles and practices will need to evolve. Here are some trends shaping the future of data governance:
- AI and Machine Learning in Governance:
- Automated data quality checks and corrections
- AI-powered metadata generation and management
- Predictive analytics for data governance risk assessment
- Edge Computing and Distributed Data Governance:
- Managing data governance at the source of data generation
- Implementing governance policies across distributed systems
- Balancing local autonomy with centralized governance
- Data Democratization and Self-Service Analytics:
- Empowering users with governed access to data
- Implementing guardrails for self-service data usage
- Balancing data access with governance requirements
- Privacy-Enhancing Technologies (PETs):
- Implementing advanced data anonymization techniques
- Using secure multi-party computation for privacy-preserving analytics
- Adopting zero-knowledge proofs for secure data verification
- Blockchain for Data Governance:
- Using distributed ledger technology for immutable audit trails
- Implementing smart contracts for automated policy enforcement
- Enhancing data provenance tracking
- Cloud-Native Data Governance:
- Adapting governance practices for cloud environments
- Implementing cloud-specific data security and privacy measures
- Leveraging cloud services for scalable governance solutions
- Data Ethics and Responsible AI:
- Incorporating ethical considerations into data governance frameworks
- Developing governance practices for AI model transparency and fairness
- Implementing mechanisms for algorithmic accountability
Wrapping Up: The Transformative Power of Data Governance Principles
As we’ve explored throughout this comprehensive guide, data governance principles form the backbone of effective information management in the digital age. By embracing these guidelines, organizations can:
- Dramatically enhance data quality and reliability
- Strengthen security measures to protect against evolving threats
- Ensure ongoing compliance with complex regulatory requirements
- Significantly improve decision-making processes at all levels
- Boost overall operational efficiency and drive innovation
- Build trust with customers, partners, and stakeholders
Remember, data governance is not a one-time project but an ongoing commitment to responsible and effective data management. By continually refining your approach and staying attuned to emerging trends and technologies, you’ll be well-equipped to handle the data challenges of today and tomorrow.
Key Takeaways:
- Data governance principles provide a framework for managing data as a valuable organizational asset
- Core principles include data ownership, quality, security, compliance, accessibility, lifecycle management, and metadata management
- Successful implementation requires a structured approach, strong leadership support, and a culture of data-driven decision-making
- Overcoming challenges involves clear communication, gradual adoption, and a focus on demonstrating value
- The future of data governance will be shaped by AI, privacy-enhancing technologies, and the need for ethical data management
By mastering these data governance principles and adapting them to your organization’s unique needs, you’ll be well-positioned to unlock the full potential of your data assets and drive sustainable business success in the data-driven economy.
Next Steps: Putting Principles into Practice
To begin your data governance journey or enhance your existing practices:
- Assess your current data governance maturity
- Identify key stakeholders and form a data governance team
- Develop a tailored data governance strategy aligned with your business goals
- Start with high-impact, achievable projects to build momentum
- Invest in ongoing training and cultural change initiatives
- Regularly review and refine your data governance practices
Remember, effective data governance is a journey, not a destination. By committing to these principles and continuously improving your practices, you’ll be well-equipped to navigate the complex data landscape and drive your organization towards data-driven success.
Justin is a full-time data leadership professional and a part-time blogger.
When he’s not writing articles for Data Driven Daily, Justin is a Head of Data Strategy at a large financial institution.
He has over 12 years’ experience in Banking and Financial Services, during which he has led large data engineering and business intelligence teams, managed cloud migration programs, and spearheaded regulatory change initiatives.