In today’s data-driven business landscape, data is often considered the most valuable asset. However, data is only as valuable as its quality, accuracy, and reliability. That’s where data quality governance comes in. In this article, we’ll explore what data quality governance is, why it’s crucial for your business, and how to implement it effectively.
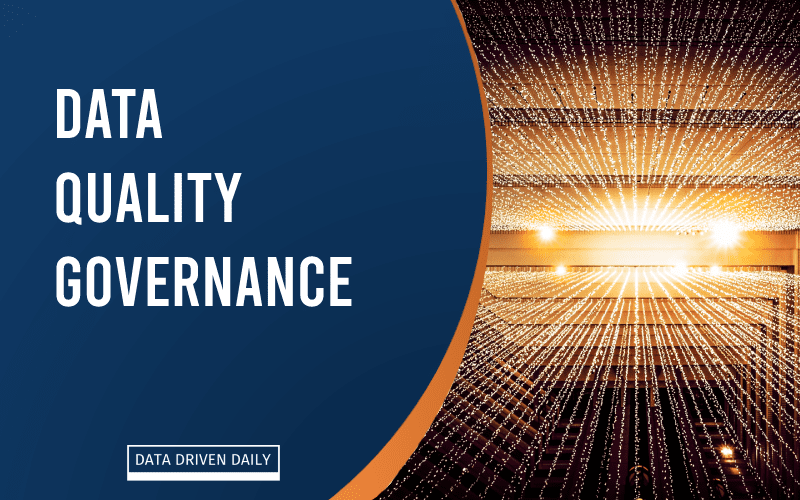
What is Data Quality Governance?
Data quality governance is the process of managing the quality, accuracy, and reliability of data across an organization. It involves establishing a framework of policies, procedures, and standards to ensure that data is consistently and accurately captured, processed, and maintained.
Why is Data Quality Governance Crucial for Your Business?
Data quality governance is essential for any data-driven business. Here are some reasons why:
Ensures Accurate Decision-Making
Data is used to inform decision-making in almost every aspect of a business, from product development to marketing to sales. If the data is inaccurate or unreliable, it can lead to flawed decisions, resulting in lost revenue, wasted resources, and reputational damage.
Maintains Compliance and Reduces Risk
Data quality governance ensures compliance with regulations such as GDPR and HIPAA. Non-compliance can result in significant fines, legal liability, and reputational damage. Data quality governance also reduces the risk of data breaches, which can have a severe impact on a business.
Improves Operational Efficiency
Data quality governance ensures that data is consistent and accurate across an organization. This consistency and accuracy make it easier to share data across different departments and systems, reducing the time and effort required to manage data.
How to Implement Data Quality Governance Effectively
Implementing data quality governance can be challenging, but it’s essential for any data-driven business. Here are some steps to help you implement data quality governance effectively:
Develop a Data Governance Strategy
A data governance strategy is a high-level plan that outlines the policies, procedures, and standards that will be used to manage data quality across an organization. It should include a framework for data quality management, data governance roles and responsibilities, and a plan for data quality monitoring and reporting.
Define Data Quality Metrics
To ensure that data is consistent and accurate across an organization, it’s essential to define data quality metrics. These metrics should be measurable, and they should align with the goals and objectives of the organization.
Establish Data Quality Standards
Data quality standards are a set of rules that define how data should be captured, processed, and maintained. These standards should be based on industry best practices and should be regularly reviewed and updated.
Implement Data Quality Monitoring
Data quality monitoring involves regularly monitoring data quality metrics to ensure that data is consistent and accurate. This monitoring should be automated wherever possible to reduce the time and effort required.
Provide Data Quality Training
Data quality governance is only effective if everyone in the organization understands the importance of data quality and how to maintain it. Providing data quality training to employees is essential to ensure that data quality governance is implemented effectively.
Data Quality Governance Framework Strategy
Implementing a data quality governance framework is essential for any organization that wants to maintain high standards of data quality. A framework should define the roles and responsibilities of data governance stakeholders, the policies and procedures for data quality management, and the tools and technologies needed to support data quality governance.
A data quality governance framework should also provide guidelines for data classification, data lineage, data profiling, and data remediation. These guidelines can help ensure that data is captured, processed, and maintained consistently and accurately across the organization.
Data Governance vs. Data Quality
Although data governance and data quality are related concepts, they are not the same thing. Data governance is the process of managing the availability, usability, integrity, and security of data. Data quality, on the other hand, is the degree to which data meets the needs of its intended use. While data governance is concerned with managing data as an asset, data quality is concerned with ensuring that the data is fit for its intended purpose.
It’s essential to understand the differences between data governance and data quality to implement an effective data quality governance strategy. Data quality governance should be seen as a subset of data governance, focused specifically on ensuring that data is accurate, reliable, and consistent.
Data Strategy and Data Quality Governance
A comprehensive data strategy is essential for any organization that wants to leverage data to gain a competitive advantage. A data strategy should define the organization’s data needs, data sources, data architecture, data management, and data governance.
Data quality governance should be an integral part of any data strategy. By ensuring that data is consistent, accurate, and reliable, organizations can make better-informed decisions, reduce risk, and improve operational efficiency.
A data strategy should also define the metrics used to measure data quality, the data quality standards, and the data quality monitoring processes. By aligning data quality governance with the overall data strategy, organizations can achieve their data-driven goals more effectively.
Challenges in Implementing Data Quality Governance
Implementing a data quality governance strategy can be challenging for many organizations. Here are some of the common challenges that organizations may face when implementing data quality governance:
Lack of Executive Support
One of the biggest challenges of implementing data quality governance is the lack of executive support. Executives may not see the value of data quality governance, or they may not understand the technical aspects of data quality management. Without executive support, it can be challenging to secure the resources and funding needed to implement a successful data quality governance strategy.
Lack of Data Quality Metrics
Another challenge of implementing data quality governance is the lack of data quality metrics. Data quality metrics are critical for measuring the effectiveness of data quality governance efforts. Without clear metrics, it can be challenging to determine whether data quality is improving or deteriorating.
Lack of Data Quality Standards
Data quality standards are a set of rules that define how data should be captured, processed, and maintained. A lack of data quality standards can lead to inconsistent data quality across the organization, making it difficult to share data and make informed decisions.
Lack of Data Quality Monitoring
Data quality monitoring involves regularly monitoring data quality metrics to ensure that data is consistent and accurate. A lack of data quality monitoring can lead to data quality issues going unnoticed, leading to flawed decisions and lost revenue.
Lack of Data Quality Training
Data quality governance is only effective if everyone in the organization understands the importance of data quality and how to maintain it. A lack of data quality training can lead to data quality issues going unnoticed or being ignored, leading to poor decision-making and reputational damage.
Additional Resources
Implementing a successful data quality governance strategy requires a thorough understanding of data governance, data quality, and related concepts. Fortunately, there are many resources available to help you develop and implement an effective data quality governance strategy.
One valuable resource is the Data Governance category on this site Data Driven Daily, where you can find informative articles, case studies, and best practices for data governance and data quality management. In particular, we recommend checking out our list of the best data governance books, which provides a comprehensive overview of the top books on data governance and related topics.
Other resources include industry associations, such as the Data Governance and Information Quality (DGIQ) Conference, which provides a forum for data governance and data quality professionals to learn, network, and share best practices. There are also online communities, such as the Data Governance Professionals Organization (DGPO), which offers training, certification, and networking opportunities for data governance professionals.
In addition to these resources, there are many books, webinars, and training courses available on data quality governance. By leveraging these resources, you can gain a deeper understanding of data governance and data quality, and develop an effective data quality governance strategy for your organization.
Conclusion
Data quality governance is crucial for any data-driven business. It ensures that data is consistent, accurate, and reliable, which is essential for informed decision-making, compliance, and operational efficiency. By developing a data governance strategy, defining data quality metrics, establishing data quality standards, implementing data quality monitoring, and providing data quality training, organizations can implement data quality governance effectively.
Implementing effective data quality governance can be challenging, but it’s essential for the success of any data-driven business. By following the steps outlined in this article, you
Justin is a full-time data leadership professional and a part-time blogger.
When he’s not writing articles for Data Driven Daily, Justin is a Head of Data Strategy at a large financial institution.
He has over 12 years’ experience in Banking and Financial Services, during which he has led large data engineering and business intelligence teams, managed cloud migration programs, and spearheaded regulatory change initiatives.