The world of data is expanding rapidly, and as a result, the fields of data science and data analytics are growing in popularity. Both play essential roles in helping organizations make data-driven decisions.
However!
They are not the same, and understanding the differences and similarities between them is crucial. In this article, we’ll explore the key differences and similarities between data science and data analytics.
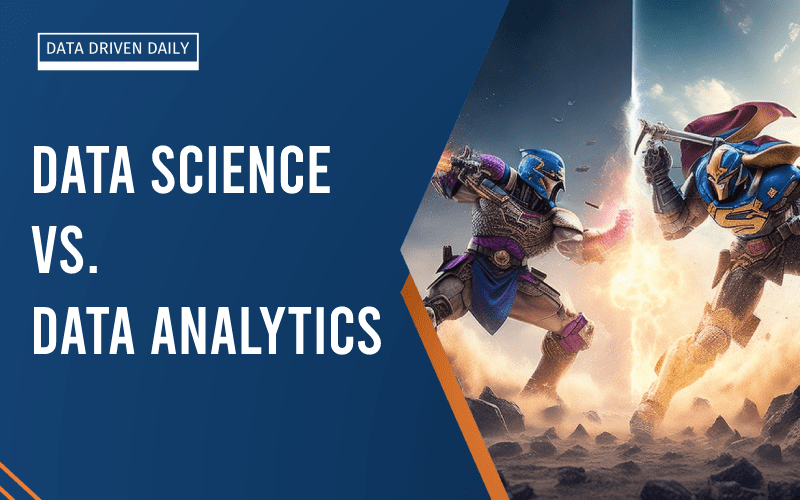
Defining Data Science
Data science is an interdisciplinary field that combines statistics, programming, and domain knowledge to extract valuable insights from large and complex datasets. Data scientists use various techniques, including machine learning, natural language processing, and data visualization, to discover hidden patterns, trends, and correlations in data. They often work with big data and unstructured data, which requires advanced tools and methods to process and analyze.
Defining Data Analytics
Data analytics is the process of analyzing, interpreting, and communicating meaningful patterns in data to support decision-making. Data analysts work primarily with structured data, using descriptive and inferential statistics to uncover insights. They often rely on tools like Excel, SQL, and Tableau to manipulate, visualize, and communicate their findings. Data analytics focuses on answering specific questions and solving well-defined problems.
Key Differences Between Data Science and Data Analytics
1. Scope and Complexity
Data science deals with larger and more complex datasets, often involving unstructured data from various sources like text, images, and videos. Data analytics, on the other hand, primarily deals with structured data in well-organized databases. Data science requires more advanced techniques and tools to process and analyze data, whereas data analytics relies on more traditional statistical methods.
2. Techniques and Methods
Data scientists use a wide range of techniques and methods, including machine learning, natural language processing, and network analysis. They often develop custom algorithms and models to extract insights from data. Data analysts use descriptive and inferential statistics to explore and summarize data, relying on tools like Excel and SQL for data manipulation and visualization.
3. Focus and Goals
Data science focuses on discovering hidden patterns, trends, and correlations in data, often with the goal of making predictions or generating recommendations. Data analytics, on the other hand, focuses on answering specific questions and solving well-defined problems. Data analysts aim to provide actionable insights to support decision-making within an organization.
4. Skills and Expertise
Data scientists need a strong background in statistics, programming (e.g., Python, R), and machine learning. They also need domain knowledge to understand the context of the data they’re working with. Data analysts need a strong foundation in statistics and data manipulation, with expertise in tools like Excel, SQL, and Tableau.
Key Similarities Between Data Science and Data Analytics
1. Data-Driven Decision-Making
Both data science and data analytics play crucial roles in helping organizations make data-driven decisions. They both involve analyzing data to extract insights that can inform business strategies and improve operations.
2. Overlapping Skills and Tools
Data scientists and data analysts often use similar tools and techniques, such as SQL, Python, R, and Tableau. Both roles require a strong foundation in statistics, as well as the ability to communicate insights effectively to stakeholders.
3. Collaboration with Stakeholders
Data scientists and data analysts both need to collaborate with business stakeholders to understand their needs, define objectives, and communicate findings. This often involves presenting insights in a clear and accessible way, using visualizations and other communication tools.
Choosing the Right Path: Data Science or Data Analytics?
If you’re considering a career in either data science or data analytics, it’s essential to understand the differences and similarities between the two fields. Data science may be a better fit if you’re interested in working with large and complex datasets, using advanced techniques like machine learning, and focusing on discovering hidden patterns and trends. Data analytics may be more suitable if you prefer working with structured data, using traditional statistical methods, and focusing on answering specific questions to support decision-making.
To further your career in either field, consider enrolling in a comprehensive training program, such as a data science bootcamp. These bootcamps can help you build the necessary skills and knowledge to excel in the world of data, whether you choose to pursue data science or data analytics.
Conclusion
Data science and data analytics are two distinct but related fields that play essential roles in helping organizations make data-driven decisions. Understanding the key differences and similarities between them can help you choose the right path for your career and ensure that you’re equipped with the skills and expertise needed to succeed in the ever-evolving world of data. By exploring the differences in scope, techniques, focus, and skills, and recognizing the overlapping aspects of data-driven decision-making, tool usage, and stakeholder collaboration, you’ll be better prepared to make an informed decision about your future in the world of data.
Ben is a full-time data leadership professional and a part-time blogger.
When he’s not writing articles for Data Driven Daily, Ben is a Head of Data Strategy at a large financial institution.
He has over 12 years’ experience in Banking and Financial Services, during which he has led large data engineering and business intelligence teams, managed cloud migration programs, and spearheaded regulatory change initiatives.