The AI landscape is moving fast, and having a solid foundation is crucial. Data powers everything AI does, but if it’s not managed well, things can go south quickly. Flawed data governance can lead to inaccurate AI models, biased results, and compliance issues that could cost you big time.
To get AI implementation right, you need to understand why data governance matters and how to put it into practice. Here’s a breakdown of why it’s essential and the steps to make sure your AI efforts are effective and responsible.
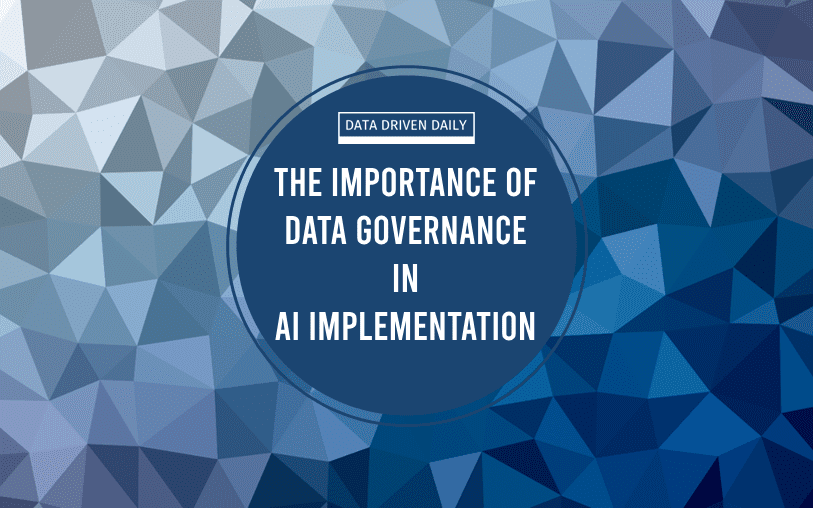
What Is Data Governance in AI?
Data governance in AI refers to the framework and practices that ensure your data is accurate, consistent, secure, and ethically handled throughout the lifecycle of your AI solutions. It covers everything from data collection to usage, privacy, and compliance. In essence, data governance establishes the rules and processes for managing data assets effectively.
Why Data Governance Is Critical in AI Implementation
Here’s the deal: Poor data governance can lead to flawed AI models, biased decision-making, and serious compliance issues. Let’s break down why data governance is non-negotiable when deploying AI.
1. Ensures Data Quality and Accuracy
AI models thrive on high-quality data. If your data is riddled with inconsistencies or errors, the output of your AI solution will be flawed. Bad data in, bad results out.
- Example: Consider an AI model used for predicting customer churn. If the data is outdated or full of errors, the predictions will be inaccurate, leading to wasted resources and poor business decisions.
- Practical Step: Implement data validation techniques and regular audits to ensure the information feeding into your AI models is up-to-date and reliable.
Quality data governance guarantees that you’re not building your AI on a shaky foundation. Instead, you’re working with trustworthy, consistent datasets that lead to actionable insights.
2. Reduces Bias and Promotes Fairness
Bias is a major problem in AI systems. When data is improperly managed, AI algorithms can reinforce existing prejudices, leading to unfair outcomes.
- Example: A facial recognition system trained on a limited, non-diverse dataset may have higher error rates for certain demographics, resulting in biased results.
- Solution: Establish governance practices that include bias detection mechanisms. Regularly test your models with diverse data sets to ensure fairness.
Data governance in AI helps mitigate bias by enforcing processes that promote inclusivity and fairness. It’s about creating transparent systems where data handling is ethical.
How to Implement Effective Data Governance in AI
Understanding the importance of data governance in AI is one thing; implementing it is another. Here’s how to do it.
1. Set Clear Data Ownership and Accountability
You need to establish who is responsible for different data assets. Define roles and responsibilities clearly to ensure accountability.
- Action Step: Create a data governance council comprising data stewards, compliance officers, and AI specialists. This council oversees data policies and ensures alignment across departments.
- Example: At a retail company, data governance might involve having specific people responsible for customer data, product data, and inventory data.
Having clear accountability structures ensures no data asset falls through the cracks.
2. Develop Robust Data Privacy and Security Policies
AI systems often require large datasets, which can include sensitive information. Ensuring privacy and data security should be a top priority.
- Best Practice: Encrypt data in transit and at rest, and use anonymization techniques when dealing with sensitive information.
- Compliance Matters: Regulations like GDPR and CCPA impose strict data governance requirements. Non-compliance can result in hefty fines.
Security measures in data governance prevent unauthorized access and keep sensitive data protected. This is essential not only for compliance but for maintaining customer trust.
Learn more about comprehensive data governance practices here.
3. Standardize Data Across the Organization
Data standardization ensures that your AI models are trained on consistent and uniform datasets, reducing the risk of errors and misinterpretations.
- Actionable Tip: Use a unified data taxonomy and metadata management practices to streamline data usage across different AI projects.
- Example: A healthcare provider implementing an AI-based diagnostic tool must standardize patient data formats to ensure accurate analysis and predictions.
Consistency across data sets is crucial. When data is standardized, AI models perform more efficiently and deliver reliable results.
4. Implement Data Lineage and Tracking
Data lineage involves tracking the origin and transformations of your data. This makes it easier to understand how data flows through your AI system, ensuring transparency.
- Why It’s Important: Knowing where your data comes from and how it has been processed helps in audits and root-cause analysis when something goes wrong.
- Practical Example: If an AI model gives unexpected results, data lineage allows you to trace back and identify where the error occurred.
Data lineage isn’t just about compliance; it’s about making your data processes more transparent and accountable.
Common Challenges in Data Governance for AI
Even the best governance frameworks face hurdles. Here are some common challenges and how to overcome them.
1. Data Silos Across Departments
Data silos create inconsistencies and make it difficult to implement uniform governance practices. When data is scattered, it becomes a nightmare to manage.
- Solution: Break down silos with centralized data platforms and cloud-based storage solutions that facilitate cross-departmental collaboration.
- Example: A manufacturing company may have separate silos for supply chain, operations, and customer data. Integrating these can streamline AI analytics and improve decision-making.
2. Balancing Data Accessibility and Security
You want your data to be easily accessible for AI model training but also secure from breaches and unauthorized access.
- Approach: Implement role-based access control and data masking techniques to maintain a balance.
- Tip: Use secure data lakes and establish policies for who can access what data and under what circumstances.
Data governance in AI is all about finding that sweet spot between making data usable for insights while keeping it secure.
The ROI of Good Data Governance
The benefits of implementing a strong data governance framework go beyond compliance. They can lead to better AI performance, more accurate insights, and higher efficiency in operations.
- Increased Model Accuracy: Clean and well-governed data leads to more reliable AI predictions.
- Regulatory Compliance: Avoid costly fines and legal headaches by adhering to data privacy laws.
- Improved Business Decisions: Quality data helps executives make more informed and impactful decisions.
When done right, data governance in AI can become a significant competitive advantage for your organization.
Future Trends in AI Data Governance
As AI continues to evolve, so do the requirements for data governance. Here are some trends to watch out for:
1. AI-Specific Data Regulations
Governments and regulatory bodies are beginning to introduce laws specific to AI data usage. Staying ahead of these regulations is crucial for companies that want to maintain a competitive edge.
2. Automated Data Governance Tools
AI-driven tools for data governance are gaining traction. These tools help automate data classification, track data lineage, and ensure compliance, making governance more efficient.
Adapting to these trends can prepare your organization for future challenges and opportunities in AI.
Final Thoughts
Data governance in AI implementation is not a box-ticking exercise. It’s a strategic necessity that can make or break your AI projects. From ensuring data quality to maintaining security and compliance, a solid governance framework is the backbone of any successful AI initiative.
Remember, investing in data governance is investing in the long-term success of your AI solutions. To maximize your efforts, you may want to explore resources like this complete guide to artificial intelligence to stay ahead of the curve.
Ben is a full-time data leadership professional and a part-time blogger.
When he’s not writing articles for Data Driven Daily, Ben is a Head of Data Strategy at a large financial institution.
He has over 14 years’ experience in Banking and Financial Services, during which he has led large data engineering and business intelligence teams, managed cloud migration programs, and spearheaded regulatory change initiatives.