Cherry-picking is a term that you may have come across in various contexts, but what does it mean in the world of data analytics?
In this comprehensive blog post, we will dive deep into the concept of cherry-picking, its implications, and how to avoid it to ensure unbiased and accurate analysis.
We will also provide you with valuable insights into how you can develop the right skills to become a data analytics expert by enrolling in one of the best data science bootcamps.
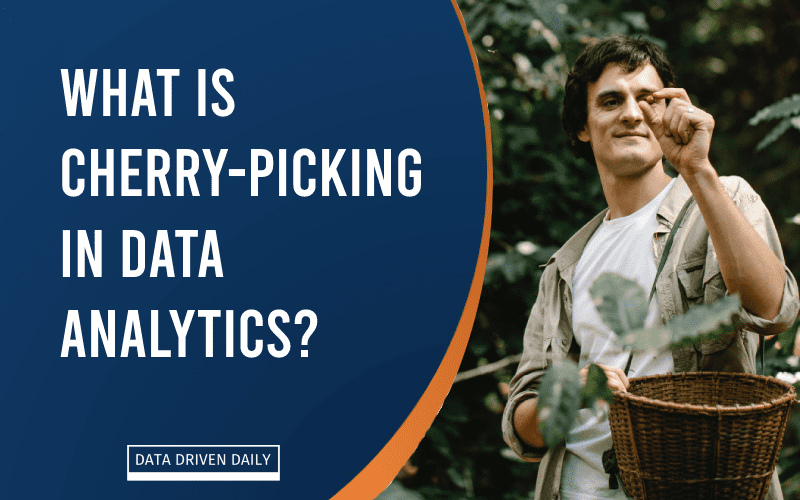
Key Takeaways |
---|
Cherry-picking in data analytics involves selectively presenting or manipulating data to support a preconceived conclusion or bias. |
This practice can lead to misleading conclusions, loss of credibility, and ineffective decision-making. |
To avoid cherry-picking, embrace transparency, pre-specify hypotheses and analysis plans, validate models, perform sensitivity analyses, and be open to contradictory evidence. |
Developing a strong foundation in data analysis techniques and best practices can help you identify and avoid cherry-picking in your work. |
- Understanding Cherry-Picking in Data Analytics
- The Implications of Cherry-Picking
- How to Avoid Cherry-Picking in Data Analytics
- Developing the Right Skills to Avoid Cherry-Picking
- Cherry-Picking in Real-World Scenarios
- The Role of Data Ethics in Avoiding Cherry-Picking
- The Importance of Data Literacy in Identifying Cherry-Picking
- Collaborative Data Analytics to Combat Cherry-Picking
- The Impact of Data Governance on Preventing Cherry-Picking
- Wrapping Up
Understanding Cherry-Picking in Data Analytics
In the context of data analytics, cherry-picking refers to the selective presentation or manipulation of data to support a preconceived conclusion or bias. It involves choosing specific data points or subsets of data that confirm one’s hypotheses while ignoring or downplaying other data that contradicts them.
Cherry-picking can lead to inaccurate or misleading conclusions, as it undermines the integrity of the data and the objectivity of the analysis.
Cherry-picking can occur in various forms, such as:
- Selective data inclusion: Choosing to include only a subset of the data that supports a particular conclusion, while excluding data that contradicts it.
- Misrepresenting data: Presenting data in a misleading manner, such as using inappropriate scales or visualization techniques that distort the true nature of the data.
- Overfitting models: Creating overly complex models that fit the training data too well, resulting in poor generalization to new, unseen data.
- P-hacking: Manipulating data or statistical tests to obtain a statistically significant result, even if it does not reflect the true underlying relationships.
The Implications of Cherry-Picking
Cherry-picking in data analytics can have several negative consequences, including:
- Misleading conclusions: By selectively presenting or manipulating data, cherry-picking can lead to false or exaggerated claims that do not reflect the true underlying relationships within the data.
- Loss of credibility: If an analyst or organization is found to have engaged in cherry-picking, they may lose their credibility, making it difficult for their work to be taken seriously in the future.
- Ineffective decision-making: Decisions based on cherry-picked data can be ineffective or even harmful, as they are not rooted in a complete and unbiased understanding of the situation.
Check out Data Analytics for Business Professionals on LinkedIn Learning which has a whole module on Cherry-picking. This is an excellent course that can help solidify some of these concepts very easily.
How to Avoid Cherry-Picking in Data Analytics
To ensure objective and accurate analysis, it is essential to avoid cherry-picking in data analytics. Here are some strategies to help you steer clear of this pitfall:
Embrace Transparency and Reproducibility
Make your data analysis process transparent by clearly documenting every step, including data collection, preprocessing, modeling, and interpretation. This enables others to review and replicate your work, ensuring that your conclusions are robust and unbiased.
Pre-specify Hypotheses and Analysis Plans
Before analyzing your data, pre-specify your hypotheses and the statistical tests or models you plan to use. This helps prevent cherry-picking by ensuring that your analysis is guided by a pre-defined plan, rather than being influenced by the data itself.
Validate Your Models
Ensure that your models are not overfitting the data by using techniques such as cross-validation, regularization, or simpler models. A well-validated model will be more likely to generalize to new data and provide accurate predictions, reducing the risk of cherry-picking.
Perform Sensitivity Analyses
Conduct sensitivity analyses to test the robustness of your conclusions under different assumptions or data subsets. This can help you identify potential biases or inconsistencies in your analysis and provide a more comprehensive understanding of the relationships within the data.
Be Open to Contradictory Evidence
Maintain an open-minded approach and be prepared to revise your hypotheses or conclusions in light of new or contradictory evidence. Embracing this mindset can help you avoid confirmation bias and ensure a more objective analysis.
Developing the Right Skills to Avoid Cherry-Picking
One way to minimize the risk of cherry-picking in data analytics is to develop a strong foundation in data analysis techniques and best practices. By enrolling in one of the best data science bootcamps, you can gain the necessary skills and knowledge to perform unbiased, accurate data analysis.
Data science bootcamps typically cover topics such as:
- Data collection and preprocessing
- Exploratory data analysis
- Statistical modeling and hypothesis testing
- Machine learning and predictive modeling
- Data visualization and communication
These bootcamps often emphasize hands-on learning through real-world projects, allowing you to develop practical experience in data analytics. By mastering these skills, you will be better equipped to identify and avoid cherry-picking in your work.
Cherry-Picking in Real-World Scenarios
To further illustrate the concept of cherry-picking in data analytics, let’s consider some hypothetical real-world scenarios:
Scenario 1: Marketing Campaign Performance
A marketing manager wants to demonstrate the success of their latest campaign. Instead of analyzing the overall performance metrics, they cherry-pick data by focusing only on the most successful channels or time periods, ignoring those that performed poorly. This selective presentation of data may paint an overly positive picture of the campaign’s effectiveness, potentially leading to misguided decisions and resource allocation.
Scenario 2: Medical Research
A pharmaceutical company conducts a clinical trial for a new drug. The researchers selectively report the positive outcomes while downplaying or ignoring negative side effects or non-significant results. This cherry-picking can mislead regulators, healthcare professionals, and patients about the safety and efficacy of the drug, with potentially serious consequences.
Scenario 3: Financial Analysis
An investment analyst is evaluating the performance of a particular stock. They cherry-pick data by focusing on specific time periods or metrics that show strong performance, while ignoring periods of poor performance or broader market trends. This biased analysis can lead to an inaccurate assessment of the stock’s potential risks and returns, potentially causing financial losses for investors who rely on the analyst’s recommendations.
The Role of Data Ethics in Avoiding Cherry-Picking
In addition to developing the right skills and following best practices, a strong commitment to data ethics can also help prevent cherry-picking in data analytics. Data ethics encompasses principles such as honesty, integrity, transparency, and accountability, which are essential for ensuring objective and accurate analysis.
When working with data, consider the following ethical guidelines:
- Be truthful in your data collection, processing, and interpretation. Do not manipulate data to fit a desired narrative or outcome.
- Be transparent about your methods and assumptions, allowing others to understand, review, and replicate your work.
- Be accountable for your actions and decisions based on data analysis, recognizing potential biases or errors and addressing them promptly.
- Consider the potential consequences of your analysis on individuals, communities, or society as a whole, and strive to minimize any negative impacts.
By incorporating data ethics into your work, you can foster a culture of trust, credibility, and responsible data use, helping to prevent cherry-picking and other biases in data analytics.
The Importance of Data Literacy in Identifying Cherry-Picking
Data literacy is the ability to understand, interpret, and communicate with data effectively. It plays a crucial role in identifying cherry-picking, as individuals with a strong foundation in data literacy are more likely to spot inconsistencies or biases in data analysis.
To improve your data literacy, focus on developing the following skills:
- Understanding data types, formats, and structures
- Knowing how to clean, preprocess, and transform data
- Familiarity with statistical concepts and techniques
- Ability to create and interpret data visualizations
- Effective communication of data insights and results
Increasing data literacy can empower individuals to make better-informed decisions, question biased or misleading analyses, and promote a more objective approach to data analytics.
Collaborative Data Analytics to Combat Cherry-Picking
Collaborative data analytics is an approach that involves working with others to collect, analyze, and interpret data. By encouraging collaboration among team members, organizations can create a system of checks and balances that helps identify and mitigate cherry-picking and other biases.
Consider implementing the following strategies to promote collaborative data analytics:
- Encourage open communication and feedback among team members, allowing for diverse perspectives and critical evaluation of data analysis.
- Establish a peer review process, where analysts’ work is reviewed and scrutinized by colleagues, fostering a culture of accountability and continuous improvement.
- Leverage tools and platforms that facilitate collaboration, such as shared data repositories, version control systems, and collaborative data analysis software.
By fostering a collaborative environment, organizations can enhance the quality and objectivity of their data analytics, reducing the risk of cherry-picking and other biases.
The Impact of Data Governance on Preventing Cherry-Picking
Data governance is a framework that defines the policies, processes, and roles for managing data within an organization. It plays a critical role in preventing cherry-picking by ensuring that data is collected, stored, and analyzed in a consistent, transparent, and accountable manner.
Effective data governance can help prevent cherry-picking by:
- Establishing clear policies and guidelines for data collection, processing, and analysis, promoting consistency and transparency across the organization.
- Defining roles and responsibilities for data management, ensuring that individuals are accountable for their actions and decisions related to data analytics.
- Implementing data quality management processes to identify and address errors, inconsistencies, or biases in the data.
- Enforcing data privacy and security measures to protect sensitive information and maintain trust among stakeholders.
By implementing a robust data governance framework, organizations can create a strong foundation for objective and accurate data analytics, minimizing the risk of cherry-picking and other biases.
Wrapping Up
Cherry-picking in data analytics is the selective presentation or manipulation of data to support a preconceived conclusion or bias. It can lead to misleading conclusions, loss of credibility, and ineffective decision-making. To avoid cherry-picking, it is crucial to embrace transparency, pre-specify hypotheses and analysis plans, validate models, perform sensitivity analyses, and be open to contradictory evidence.
Developing a strong foundation in data analysis techniques and best practices can help you identify and avoid cherry-picking in your work. By enrolling in one of the best data science bootcamps, you can gain the necessary skills and knowledge to perform unbiased, accurate data analysis and make well-informed decisions based on a complete and objective understanding of the data.
Ben is a full-time data leadership professional and a part-time blogger.
When he’s not writing articles for Data Driven Daily, Ben is a Head of Data Strategy at a large financial institution.
He has over 12 years’ experience in Banking and Financial Services, during which he has led large data engineering and business intelligence teams, managed cloud migration programs, and spearheaded regulatory change initiatives.