In the era of big data, the need for a comprehensive ecosystem of open-source software for big data management is increasingly critical.
Organizations large and small grapple with the challenges of collecting, processing, storing, and analyzing vast amounts of data.
Open-source software solutions offer a flexible, cost-effective approach to meeting these challenges. In this article, we will explore the most popular open-source tools and frameworks for big data management and how they fit together to create a cohesive ecosystem.
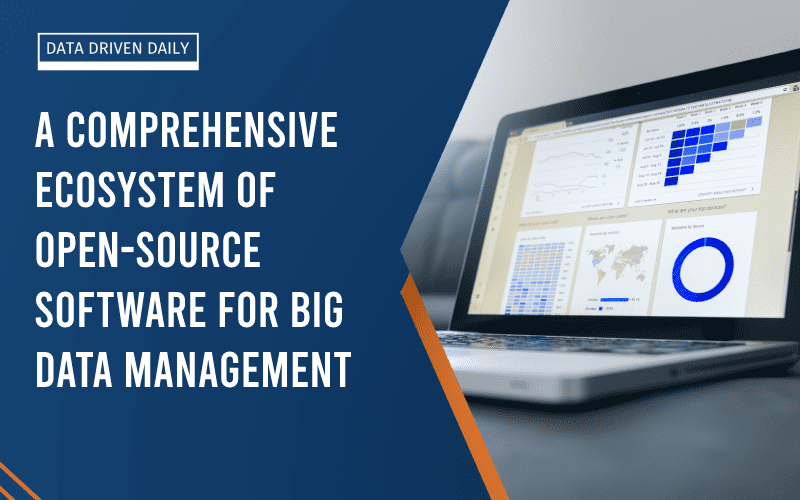
The Rise of Open-Source Software in Big Data Management
As the volume, variety, and velocity of data continue to grow, so does the need for efficient and scalable data management solutions. Traditional proprietary tools often come with high licensing costs and may lack the flexibility required to adapt to the ever-changing big data landscape.
In contrast, open-source software offers a more versatile and cost-effective alternative. With a global community of developers contributing to the code base, open-source tools benefit from rapid innovation and continuous improvement. This collaborative approach enables organizations to customize solutions to their specific needs while avoiding vendor lock-in.
Key Components of a Comprehensive Ecosystem
To create a comprehensive ecosystem of open-source software for big data management, we must consider the various stages of the data lifecycle, from ingestion and storage to processing, analysis, and visualization. The following sections will delve into the most popular tools and frameworks within each of these stages.
Data Ingestion and Integration
Data ingestion involves acquiring data from various sources, such as sensors, logs, databases, and APIs, and integrating it into a central storage system. Key open-source tools for data ingestion and integration include:
- Apache NiFi: A powerful data flow management tool that supports data routing, transformation, and enrichment, enabling users to design, schedule, and monitor data flows.
- Logstash: A versatile data collection and processing engine, Logstash is part of the Elastic Stack (ELK) and can ingest data from multiple sources, transforming and enriching it before sending it to Elasticsearch for storage and analysis.
- Apache Kafka: A high-throughput, distributed messaging system, Kafka is designed for real-time data streaming and can handle millions of events per second.
Data Storage
Once ingested, data must be stored in a way that facilitates efficient retrieval and processing. Key open-source storage technologies include:
- Hadoop Distributed File System (HDFS): A foundational component of the Hadoop ecosystem, HDFS is a scalable, distributed file system designed for large-scale data storage and processing.
- Apache Cassandra: A highly scalable, distributed NoSQL database, Cassandra is designed for managing large amounts of structured and semi-structured data across many commodity servers.
- Elasticsearch: Part of the Elastic Stack, Elasticsearch is a distributed, full-text search and analytics engine that excels at handling large volumes of structured and unstructured data.
Data Processing and Analysis
Data processing and analysis involve transforming, aggregating, and exploring data to derive insights and make data-driven decisions. Key open-source tools for data processing and analysis include:
- Apache Hadoop: A comprehensive ecosystem of open-source software for big data management, Hadoop includes HDFS for storage, YARN for resource management, and MapReduce for distributed data processing.
- Apache Spark: An advanced data processing framework, Spark offers in-memory processing, support for various programming languages, and built-in libraries for machine learning, graph processing, and stream processing.
- Apache Flink: A powerful stream processing framework, Flink excels at processing real-time data streams and offers advanced features like event time processing and stateful computations.
Visualizing and reporting data is essential for making it accessible and actionable to stakeholders. Open-source tools for data visualization and reporting include:
- Kibana: Part of the Elastic Stack, Kibana is a flexible data visualization and exploration platform that provides real-time, interactive dashboards and reporting capabilities.
- Grafana: A popular open-source analytics and monitoring platform, Grafana supports various data sources, including Elasticsearch, InfluxDB, and Prometheus, and offers customizable dashboards and alerting features.
- Apache Superset: A modern data exploration and visualization platform, Superset supports a wide range of data sources and offers rich, interactive visualizations, customizable dashboards, and SQL-based exploration.
The Importance of Integration and Interoperability
A comprehensive ecosystem of open-source software for big data management requires seamless integration and interoperability among its various components. Integration ensures that data flows smoothly and efficiently between ingestion, storage, processing, analysis, and visualization stages, while interoperability ensures that tools and frameworks can work together effectively, regardless of their specific data formats, APIs, or protocols.
To achieve this level of integration and interoperability, many open-source big data projects adopt common standards and interfaces, such as the Hadoop ecosystem’s support for HDFS and YARN, or the Elastic Stack’s use of the Elasticsearch API. In addition, some projects offer connectors or integrations with other popular tools, enabling users to build a cohesive big data management solution that leverages the best of each component.
The Role of Cloud-Based Services and Platforms
While deploying and managing an open-source big data stack on-premises can be complex and resource-intensive, cloud-based services and platforms offer a more accessible and scalable alternative. Major cloud providers, such as Amazon Web Services, Google Cloud Platform, and Microsoft Azure, offer managed services for popular open-source big data tools, including Hadoop, Spark, Elasticsearch, and more.
These managed services simplify the deployment, scaling, and maintenance of big data infrastructure, enabling organizations to focus on deriving insights and value from their data, rather than managing the underlying infrastructure. In addition, cloud providers often offer tight integration between their big data services and other cloud-based tools, such as machine learning, data warehousing, and analytics platforms, further enhancing the capabilities of a comprehensive ecosystem of open-source software for big data management.
Wrapping Things Up
A comprehensive ecosystem of open-source software for big data management offers organizations a flexible, cost-effective, and scalable solution to the challenges posed by the ever-growing volume, variety, and velocity of data. By leveraging the most popular open-source tools and frameworks for data ingestion, storage, processing, analysis, and visualization, and ensuring seamless integration and interoperability among these components, organizations can build a cohesive big data management solution tailored to their specific needs.
As the big data landscape continues to evolve, the role of open-source software will only grow more critical, driven by the rapid innovation and continuous improvement made possible by the global developer community. By embracing open-source solutions, organizations can stay agile and competitive in the era of big data, harnessing the power of their data to drive better decision-making and create new opportunities for growth.
Ben is a full-time data leadership professional and a part-time blogger.
When he’s not writing articles for Data Driven Daily, Ben is a Head of Data Strategy at a large financial institution.
He has over 12 years’ experience in Banking and Financial Services, during which he has led large data engineering and business intelligence teams, managed cloud migration programs, and spearheaded regulatory change initiatives.