Picture a world where businesses can analyze massive amounts of data in minutes, not hours or days, and make informed decisions based on these analyses.
Welcome to the world of AI data analytics!
This powerful combination of artificial intelligence (AI) and data analytics is revolutionizing the way we process, understand, and leverage data.
In this article, we’ll explore what AI data analytics is, its applications in various industries, and how it’s transforming data analysis with real-world examples.
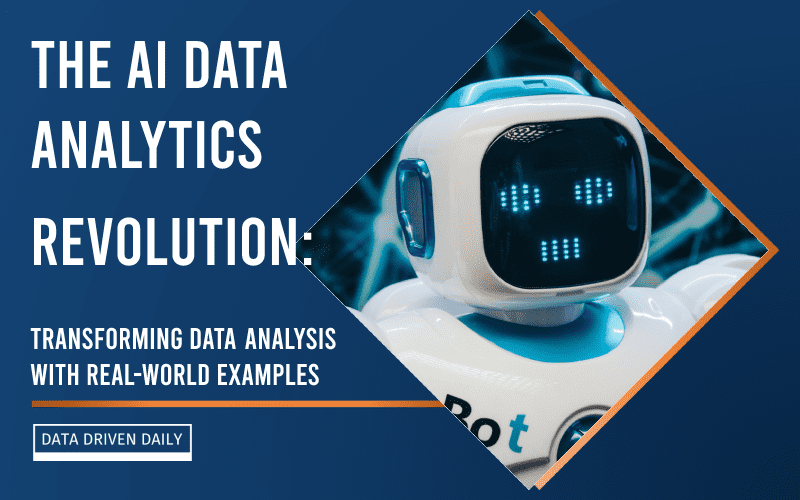
Key Takeaways |
---|
AI data analytics revolutionizes industries |
AutoML democratizes machine learning |
Explainable AI ensures transparency |
Edge AI enables real-time data analysis |
Importance of AI ethics and regulation |
GPT-4’s role in conversational data analytics |
What is AI Data Analytics?
AI data analytics refers to the use of AI algorithms and techniques, such as machine learning and natural language processing, to analyze and extract insights from large datasets.
This approach goes beyond traditional data analytics methods by automating the process of finding patterns, trends, and relationships in the data, making it faster and more efficient.
For those interested in pursuing a career in AI data analytics, it’s essential to have a solid foundation in data science and AI. One way to acquire these skills is by enrolling in one of the best data science bootcamps or obtaining a data science certificate.
Real-Life Applications of AI Data Analytics
Healthcare
AI data analytics is transforming the healthcare industry by enabling more accurate diagnoses and personalized treatment plans.
For example, AI algorithms can analyze medical images, such as X-rays and MRIs, to identify patterns that may indicate a particular condition. This helps doctors make better decisions, improving patient outcomes.
Finance
Financial institutions use AI data analytics to detect fraudulent transactions, assess credit risk, and optimize investment strategies. By analyzing vast amounts of historical data, AI algorithms can identify patterns that signal potential fraud or predict future market trends, allowing companies to make more informed decisions and minimize risk.
Retail
Retailers are leveraging AI data analytics to understand customer preferences, personalize marketing campaigns, and optimize pricing strategies. By analyzing customer data, such as purchase history and browsing behavior, AI algorithms can generate product recommendations tailored to individual preferences, driving sales and increasing customer satisfaction.
Manufacturing
AI data analytics is helping manufacturers optimize production processes, reduce waste, and improve quality control. By analyzing data from sensors and equipment, AI algorithms can predict when a machine may fail, allowing for proactive maintenance and reducing downtime. Additionally, AI-powered analytics can identify inefficiencies in production processes, leading to cost savings and increased productivity.
Real-World Examples of AI Data Analytics in Action
Example 1: Cancer Detection
One of the most promising applications of AI data analytics is in cancer detection. For instance, researchers at Stanford University developed an AI algorithm that can analyze skin cancer images with remarkable accuracy.
By training the algorithm on a large dataset of dermatoscopic images, the AI system can identify different types of skin cancer with an accuracy comparable to human dermatologists, potentially saving lives and reducing healthcare costs.
Example 2: Optimizing Energy Consumption
AI data analytics is also being used to optimize energy consumption in smart homes and buildings. By analyzing data from various sensors, such as temperature, humidity, and occupancy, AI algorithms can adjust heating and cooling systems in real-time to maximize energy efficiency, reducing both energy consumption and costs.
Example 3: Enhancing Customer Service
AI-powered chatbots are becoming increasingly popular in customer service, thanks to AI data analytics. These chatbots can analyze customer inquiries, determine the best way to address the issue, and provide accurate and timely responses. This not only improves customer satisfaction but also frees up human agents to focus on more complex tasks.
GPT-4: The Future of AI-Powered Conversational Agents in Data Analytics
One of the most exciting advancements in the field of AI data analytics is the development of sophisticated conversational agents, such as GPT-4.
GPT-4, the latest version of OpenAI’s Generative Pre-trained Transformer, is a cutting-edge AI model that has shown remarkable capabilities in natural language understanding, generation, and even conversational skills.
The potential of GPT-4 in AI data analytics is immense, with applications ranging from advanced chatbots to natural language processing tasks.
GPT-4 and AI Data Analytics
GPT-4’s vast knowledge base and advanced natural language capabilities make it an excellent candidate for AI data analytics applications. By leveraging GPT-4, businesses can create more engaging and human-like conversational agents capable of understanding complex queries and providing relevant, insightful responses. This can help businesses streamline their customer support, gather valuable user feedback, and even automate certain data analysis tasks.
For instance, GPT-4 can be used to create AI-powered chatbots that interact with users to help them navigate and understand complex datasets. These chatbots can understand and interpret user queries, perform data analysis tasks, and present the results in a conversational and easy-to-understand manner. This can save time and resources by automating routine data analysis tasks while providing users with a more intuitive and engaging experience.
GPT-4 in Business Intelligence and Data Visualization
GPT-4’s advanced natural language capabilities also make it a valuable tool in business intelligence and data visualization. By incorporating GPT-4 into data analytics platforms, businesses can enable users to interact with their data using natural language queries, making it easier for non-technical users to explore and analyze data without the need for specialized training.
For example, GPT-4 can be integrated with data visualization tools to generate insightful narratives and summaries based on the visualizations, providing users with a more comprehensive understanding of the data being presented. This can help businesses make data-driven decisions more efficiently and effectively, as users can quickly grasp the insights and trends hidden within their data.
Challenges and Considerations
While GPT-4 offers immense potential in AI data analytics, there are also challenges and considerations to address. As GPT-4 is a powerful language model, it may sometimes generate plausible-sounding but incorrect or misleading information. Ensuring the accuracy and reliability of GPT-4’s responses is crucial, particularly when applied to data analysis tasks.
Additionally, ethical considerations around AI data analytics, including privacy and potential biases, must be addressed. Businesses should establish guidelines and best practices for using GPT-4 and similar AI models to ensure that they are used responsibly and ethically.
The Future of AI Data Analytics
As technology continues to advance, AI data analytics will only become more powerful and essential across various industries. The future of AI data analytics is promising, with several emerging trends and developments set to shape its evolution.
Automated Machine Learning (AutoML)
AutoML is an emerging field within AI data analytics that aims to simplify the process of building, training, and deploying machine learning models. With AutoML, even those without extensive data science expertise can leverage AI algorithms to analyze data, making AI data analytics more accessible to a wider audience.
Explainable AI
As AI algorithms become more complex and capable, it’s crucial to understand the decision-making process behind their predictions and recommendations. Explainable AI seeks to make AI algorithms more transparent, enabling users to understand the reasoning behind the AI system’s outputs. This will not only improve trust in AI but also help identify potential biases and inaccuracies in the algorithms.
Edge AI
Edge AI refers to the deployment of AI algorithms on local devices, such as smartphones or IoT devices, rather than in the cloud. This allows for real-time data analysis with lower latency, as the data doesn’t need to be sent to the cloud for processing. Edge AI can also enhance data privacy, as sensitive information can be processed locally without being transmitted to remote servers.
AI Ethics and Regulation
As AI data analytics becomes more widely adopted, concerns regarding ethical considerations and potential misuse of the technology are likely to increase. Governments and industry organizations will need to develop guidelines and regulations to ensure that AI data analytics is used responsibly and ethically, protecting individual privacy and preventing discrimination or bias.
Conclusion
AI data analytics is revolutionizing the way businesses and organizations leverage data to make informed decisions, optimize processes, and enhance customer experiences. By using a conversational tone and real-life examples, we’ve highlighted the potential of AI data analytics across various industries and explored its future developments. As AI data analytics continues to advance, it will become an even more critical tool for businesses and individuals seeking to harness the power of data. To stay competitive in this rapidly evolving field, consider investing in your education by enrolling in one of the best data science bootcamps or obtaining a data science certificate.
Ben is a full-time data leadership professional and a part-time blogger.
When he’s not writing articles for Data Driven Daily, Ben is a Head of Data Strategy at a large financial institution.
He has over 12 years’ experience in Banking and Financial Services, during which he has led large data engineering and business intelligence teams, managed cloud migration programs, and spearheaded regulatory change initiatives.