Embarking on a data science internship is a pivotal step for aspiring data scientists. These internships offer a practical, hands-on experience that is crucial for understanding the intricacies of the field. They provide a unique opportunity to apply theoretical knowledge to real-world problems, bridging the gap between academic learning and professional application.
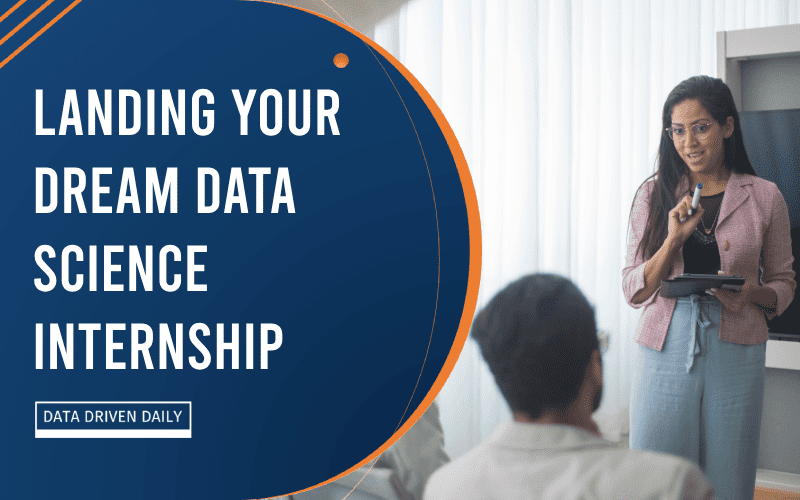
Why Pursue a Data Science Internship?
Internships in data science are more than just temporary job positions; they are a launching pad for a successful career. They allow you to immerse yourself in the data-driven decision-making process, work with seasoned professionals, and gain insights into the industry’s operational dynamics. The experience gained can be invaluable, offering a glimpse into the day-to-day responsibilities of a data scientist and helping to build a robust professional network.
How to Find the Right Data Science Internship
Identify Your Interests
Data science is a broad field, encompassing various specialties like machine learning, data visualization, and predictive modeling. Identifying your interest area can help you target your internship search more effectively.
Leverage Online Resources
Numerous platforms list internship opportunities in data science. Websites like Indeed, LinkedIn, and Glassdoor can be excellent starting points. Regularly visiting company websites and career pages can also unveil opportunities in data science roles.
Networking
Don’t underestimate the power of networking. Engaging with professionals through conferences, seminars, or even social media can open doors to internship opportunities. Professional associations related to data science also often post job listings or have forums where opportunities are shared.
Building a Standout Profile for Data Science Internship Applications
Crafting a profile that captures attention is crucial when competing for data science internships. Your resume and online presence should clearly articulate your skills, projects, and passion for data science.
Crafting an Engaging Resume
Your resume should highlight relevant coursework, projects, and any experience that showcases your data science skills. Don’t just list technologies and methods; illustrate how you’ve applied them in real or simulated projects.
Leveraging LinkedIn
LinkedIn is an indispensable tool. Ensure your profile is up-to-date, detailing your educational background, projects, and any relevant experiences. Engaging with data science content and connecting with professionals in the field can also enhance your visibility and chances of finding a suitable internship.
Building a Portfolio
A portfolio can differentiate you from other candidates. It should include a selection of your best work, ideally demonstrating a range of skills and interests within data science. Websites like GitHub or personal blogs can serve as excellent platforms to showcase your portfolio.
Navigating the Data Science Internship Interview Process
Securing an interview is a significant milestone in obtaining a data science internship. Preparation is key to navigating this stage successfully.
Understanding Interview Formats
Be prepared for different types of interviews, ranging from technical screenings to behavioral interviews. Technical interviews may involve coding challenges, statistical questions, or case studies to test your problem-solving skills in a data science context.
Preparing for Technical Questions
Familiarize yourself with common data science algorithms, statistical methods, and programming questions. Practice problem-solving on platforms like LeetCode or HackerRank to hone your skills.
Demonstrating Soft Skills
While technical prowess is crucial, don’t underestimate the importance of soft skills. Clear communication, teamwork, and problem-solving are highly valued in data science roles. Be ready to discuss how you’ve used these skills in past experiences.
Making the Most of Your Data Science Internship
Engage Actively
Show enthusiasm and willingness to learn. Engaging actively with your tasks, asking questions, and demonstrating curiosity can set you apart as an intern.
Work on Real Projects
The core of a data science internship should involve working on tangible projects. This exposure is critical as it allows you to understand how data science projects are conceptualized, developed, and deployed in a real-world setting.
Seek Feedback
Regular feedback from your supervisors can be incredibly beneficial. It can provide insights into your performance and areas where you can improve.
Transitioning from an Intern to a Full-Time Role
Showcase Your Achievements
At the end of your internship, having a portfolio or a compilation of the projects you’ve worked on can be a significant asset. It demonstrates your capability and hands-on experience to potential employers.
Maintain Professional Relationships
The connections you make during your internship can be valuable throughout your career. Maintaining these relationships can lead to future job opportunities or collaborations.
Reflect and Learn
Post-internship, take time to reflect on what you’ve learned and how you can apply this knowledge moving forward. Continuous learning is key in the ever-adapting field of data science.
Final Thoughts
A data science internship is a vital stepping stone in the journey to becoming a proficient data scientist. It offers a rare glimpse into the industry’s workings, helps build essential skills, and establishes a foundation for a promising career. By choosing the right internship, engaging fully during the experience, and leveraging the learnings post-internship, you can pave the way for a successful career in data science.
By following these guidelines and approaching your data science internship with enthusiasm and a willingness to learn, you can maximize the opportunity and set the stage for a successful career in the field.
Ben is a full-time data leadership professional and a part-time blogger.
When he’s not writing articles for Data Driven Daily, Ben is a Head of Data Strategy at a large financial institution.
He has over 14 years’ experience in Banking and Financial Services, during which he has led large data engineering and business intelligence teams, managed cloud migration programs, and spearheaded regulatory change initiatives.